The benefits of collecting EEG neuroimaging insights in clinical trials.
The benefits of collecting EEG neuroimaging insights in clinical trials
Jan 30, 2023 • 5 min read • Download article
In clinical trials, brain monitoring thanks to electroencephalography (EEG) is facilitating the measurement of complex clinical endpoints that previously would have only been possible at the research clinical step. The virtuous circle of health data, bidirectional clinical data and patient care data will facilitate the improvement of quality services and alleviate the global healthcare burden.
Latest AI-powered brainwave monitoring combine artificial intelligence and neuroscience, to record, analyze and interpret in real time the electrical signals of the brain, giving direct insights into brain pathological oscillations and emotional processes in clinical studies[1][2].
This longitudinal brain data combined with user-friendly platforms hold the potential to change the way we diagnose, treat and care for people living with neurological conditions. EEG as a biomarker provides safe, objective and repeatable data enabling a deeper understanding of the pharmacological effects of the drug early in its development.
AI powered brainwave monitoring.
In a world where there is a growing need to expand healthcare access, brain monitoring technology offers solutions to identify critical biomarkers and enable personalized patient care at affordable prices. Artificial Intelligence (AI) and Machine Learning (ML) have a crucial role to play in the burgeoning discipline of digital medicine.
The global remote patient monitoring market is projected to reach 175.2 Billion USD by 2027 from 53.6 Billion USD in 2022, at a compact annual growth rate of 26.7%[3]. The number of registered clinical trials within the past decade has also increased drastically. Pharmaceutical Research and Manufacturers of America estimated the cost at 10 Billion USD spent on 1680 phase 3 clinical trials comprising over 600,000 patients[4].
Statistical significance is no longer enough. It is time to focus on the clinical significance of a trial — whether it has a noticeable impact on the daily lives of patients. Each of the facets of protocol design and successive trial planning offers opportunities for improvement. In a review of 57 dementia drug trials, researchers found that less than half (46%) discussed the clinical significance of their results.
The most commonly cited measures of clinical significance in these trials were the 4-point change in the Alzheimer’s Disease Assessment Scale-Cognitive Subscale (ADAS-Cog) and changes on global scales. This self assessment scale does not constitute sufficient weight to change the course of a study[5]. The majority of measures of clinical significance are opinion based and are therefore limited by their subjective aspect. Self assessment scales must be completed with objective measures that solidify findings and take clinical trials to the next stage.
Patient centric AI.
Patient centric AI means using robust analytical methods to parse multi-dimensional data more thoroughly and in a way that would be all but impossible via simple human calculations in order to formulate more personalized treatments and prognosis solutions[6]. This type of data recordings are coupled to a powerful data processing engine to obtain user-friendly medical dashboards.
This available pertinent data will not only enable remote patient monitoring services, it will also provide healthcare professionals the ability to make personalized clinical decisions, at scale. These computational models are at the basis of digital medicine and have the potential to paint a high resolution picture of an individual’s unique neurological condition which could perhaps aid in the early diagnosis of neurodegenerative diseases or at least improve the longitudinal patient monitoring.
Electroencephalography is also facilitating the measurement of complex clinical endpoints. Monitoring brain states outside the clinic is now possible with EEG devices enabling new ways to assess patient reported outcomes. Subjective and quantitative human data are different in nature. One is declarative and informs us about the subjective perception, the other is quantitative and informs us about the physiological responses.
Crossing this information to better decode a behavior or even better stratify the populations recorded is becoming essential. By using physiological data (i.e. EEG) with psychometric data (i.e. self-report) – new AI powered systems can process new levels of information. Crossing brain wave monitoring EEG data with patients’ reported outcomes will increase industry innovation and pave the way for an efficient path for patients to access safe, effective new treatments.
A clear path to clinical applications.
Electroencephalography has gained prominence as an ideal biomarker as it is continuous, objective, repeatable, reproducible, sensitive, non-invasive and highly translatable from non-clinical data[7]. EEG provides safe neuromarkers in phase 1 and 2 of a clinical trial on a smaller number of patients but with a higher density of electrodes enabling a deeper understanding of the pharmacological effects of the drug early in its development.
To solidify accuracy of treatment findings in phase 3 and 4, EEG monitoring enables follow-up of a much greater quantity of patients. EEG is one of the only methods that can pass through the different scales of a clinical trial with a very high level of precision and at an affordable price. This technology may ultimately reduce clinical trial duration and sample size by enrolling better characterized study participants as soon as phase 1, allowing faster and more accurate demonstration of a potential drug effect.
EEG is already being used as a clinical endpoint, as is the case for the following recent study “Predictive Power of Cognitive Biomarkers in Neurodegenerative Disease Drug Development” (Olichney & al., 2022[8]). Through the application of standardized EEG, P300 Event-Related Potential (ERP P300) can be reliably measured. ERPs and evoked potentials (EPs) are one of many useful applications of electroencephalography, in that they capture averaged brain responses that are task-based and time-locked and thus, are associated with specific sensory and motor EPs or cognitive events. The P300 waveform objectively measures large-scale neuronal network functioning and working memory processes. Increased ERP P300 latency has been reported throughout the literature in disorders of cognition, supporting the potential utility of ERP P300 as a biomarker in many neurological and neuropsychiatric disorders, including Alzheimer’s Disease (AD). Specifically, evidence presented here supports ERP P300 latency as a quantitative, unbiased measure for detecting changes in cognition in patients with AD dementia through the progression from mild to moderate cognitive impairment and after drug treatment. This technology holds the potential to change the way we diagnose, treat and care for people living with deadly neurological conditions.

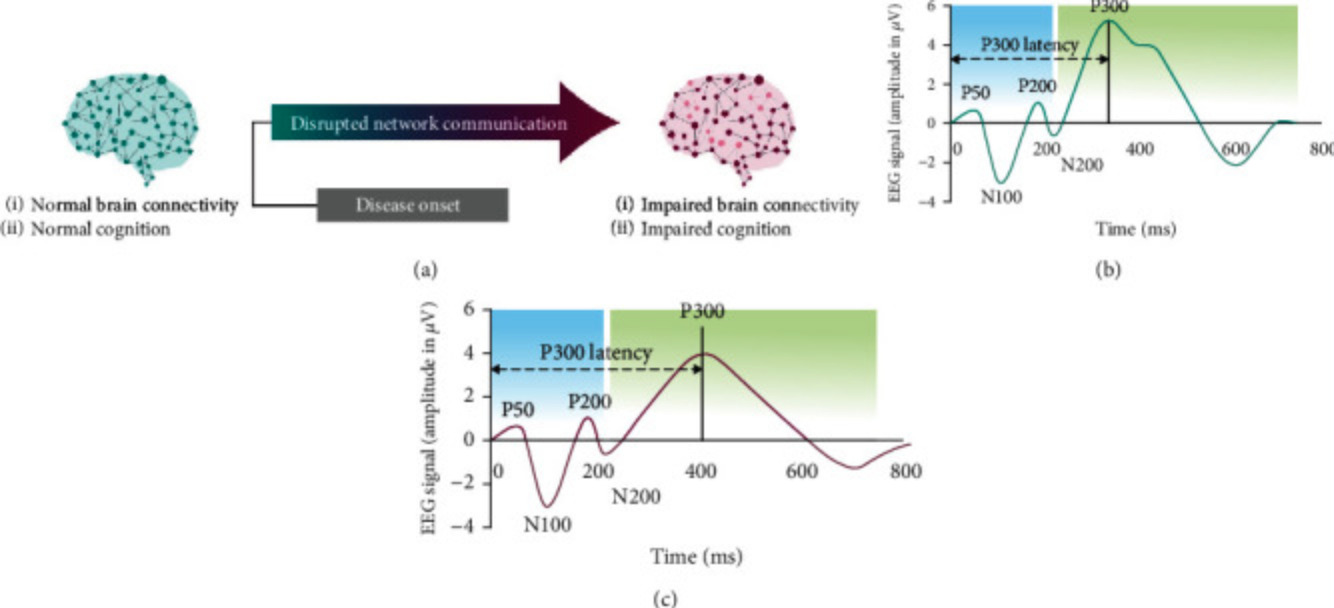
In this figure, the shape ERP waveform is representative of neurotypical brain activity and normal cognition (b). A characteristic response is detected 300 ms after presentation of stimuli (P300). Early in disease onset, and usually prior to the diagnosis of AD, neural network communication is disrupted, leading to impaired brain connectivity and impaired cognition. This change in cognition can be detected as a change in the peak and/or latency (time to onset of peak) of the P300 response to stimuli (c).
There are other EEG features as powerful as the P300 to follow other parameters. Spectral analysis of power or network connectivity have great potential in the health sector of tomorrow[9].
In conclusion.
In a world where there is a growing need to expand healthcare access, EEG technology offers a solution to identify stable biomarkers and create personalized patient care at affordable prices. It is essential today to measure the clinical significance of a treatment effect — whether it has a real noticeable effect on the daily life of patients. EEG monitoring tools explore neuronal interactions at the network level identifying shared patterns of brain activity across large sample populations.
The more we use these tools in clinical trials, the more robust and scalable will be the results; putting new effective treatments on the market with great accuracy and at affordable prices. Digitalized neuromarkers as EEG clinical endpoints are a key feature in drug development today. We are on the path to understanding and preventing how human cognition may degrade with aging and diseases. We are only at the beginning of what can be a revolution in the way we treat neuronal and psychiatric care.
References.
[1] Babiloni, C., & al. (2020). What electrophysiology tells us about Alzheimer’s disease: a window into the synchronization and connectivity of brain neurons. Neurobiology of aging, 85, 58–73. https://doi.org/10.1016/j.neurobiolaging.2019.09.008
[2] Guillon, J., Attal, Y., Colliot, O., Dubois, B., Schwartz, D., Chavez, M. & De Vico Fallani, F. (2017). Loss of brain inter-frequency hubs in Alzheimer’s disease. Sci Rep, 7, 10879. https://doi.org/10.1038/s41598-017-07846-w
[3] Remote Patient Monitoring Market (2022). MarketsandMarkets. https://www.marketsandmarkets.com/Market-Reports/remote-patient-monitoring-market-77155492.html
[4] Fogel D. B. (2018). Factors associated with clinical trials that fail and opportunities for improving the likelihood of success: A review. Contemporary clinical trials communications, 11, 156–164. https://doi.org/10.1016/j.conctc.2018.08.001
[5] Heneghan, C., Goldacre, B. & Mahtani, K.R. (2017). Why clinical trial outcomes fail to translate into benefits for patients. Trials, 18, 122. https://doi.org/10.1186/s13063-017-1870-2
[6] Belachew, S. (2022, March 01). How patient-oriented AI solutions are transforming care for neurodegenerative disease. Biogen. https://www.biogen.com/stories/patient-oriented-ai-solutions.html
[7] Biotrial (n. d.). EEG. https://www.biotrial.com/services/core-lab/eeg.html
[8] Olichney, J., Xia, J., Church, K. J., & Moebius, H. J. (2022). Predictive Power of Cognitive Biomarkers in Neurodegenerative Disease Drug Development: Utility of the P300 Event-Related Potential. Neural plasticity, 2022, 2104880. https://doi.org/10.1155/2022/2104880
[9] Guillon, J., Chavez, M., Battiston, F., Attal, Y., La Corte, V., Thiebaut de Schotten, M., Dubois, B., Schwartz, D., Colliot, O., & De Vico Fallani, F. (2019). Disrupted core-periphery structure of multimodal brain networks in Alzheimer’s disease. Network Neuroscience, 3(2), 635–652. https://doi.org/10.1162/netn_a_00087
Related Articles
Get in touch
We are glad you are interested in reaching out to us. Whether you have a question about our products or want to give us feedback, we are here to help.